Learning GAN-based Foveated Reconstruction to Recover Perceptually Important Image Features
1Università della Svizzera italiana 2West Pomeranian University of Technology 3University of Groningen 4Max Planck Institute for Informatics
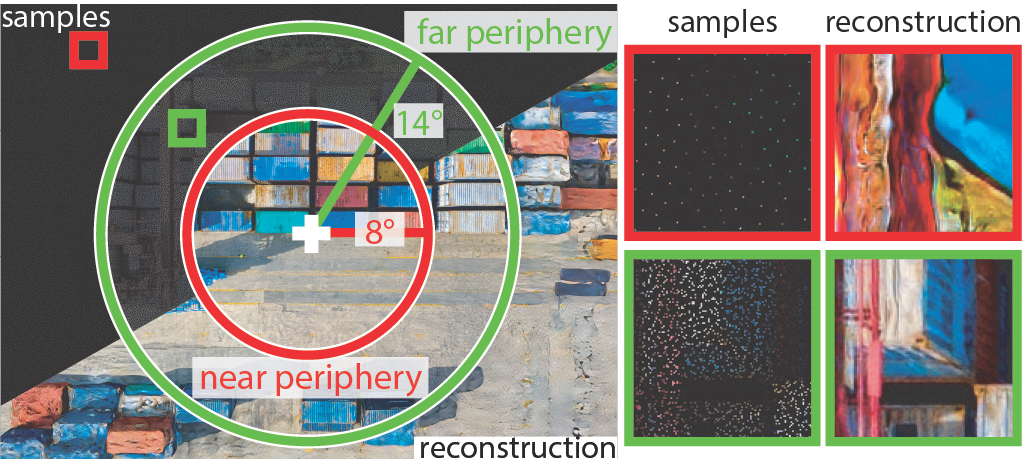
Abstract:
A foveated image can be entirely reconstructed from a sparse set of samples distributed according to the retinal sensitivity of the human visual system, which rapidly decreases with increasing eccentricity. The use of Generative Adversarial Networks has recently been shown to be a promising solution for such a task, as they can successfully hallucinate missing image information. As in the case of other supervised learning approaches, the definition of the loss function and the training strategy heavily influence the quality of the output. In this work,we consider the problem of efficiently guiding the training of foveated reconstruction techniques such that they are more aware of the capabilities and limitations of the human visual system, and thus can reconstruct visually important image features. Our primary goal is to make the training procedure less sensitive to distortions that humans cannot detect and focus on penalizing perceptually important artifacts. Given the nature of GAN-based solutions, we focus on the sensitivity of human vision to hallucination in case of input samples with different densities. We propose psychophysical experiments, a dataset, and a procedure for training foveated image reconstruction. The proposed strategy renders the generator network flexible by penalizing only perceptually important deviations in the output. As a result, the method emphasized the recovery of perceptually important image features. We evaluated our strategy and compared it with alternative solutions by using a newly trained objective metric, a recent foveated video quality metric, and user experiments. Our evaluations revealed significant improvements in the perceived image reconstruction quality compared with the standard GAN-based training approach.
Sample results:
The displayed images are an extended version of Figures 1, 7 of the paper. Hovering the mouse on the image, it is possible to compare the four methods (L2, L2 ours, LPIPS, LPIPS ours).
The area under the magnifier (white square) is displayed for each method on the right of the full resolution image together with the zoomed version and the standard foveation.
The red and green rings are the boundaries between full resolution-near periphery and near periphery-far periphery, respectively. These three regions are blended in the boundary with a smooth transition.
You may want to click on each square to display the full image.
Please use the following menu to change the displayed image.
Mouse over the image:
Acknowledgements:
This project has received funding from the European Research Council (ERC) under the European Union's Horizon 2020 research and innovation program (grant agreement N◦ 804226 PERDY).